Voice-based analysis may help differentiate FA from other diseases
Machine learning could be used for vocal analysis in limited healthcare setting
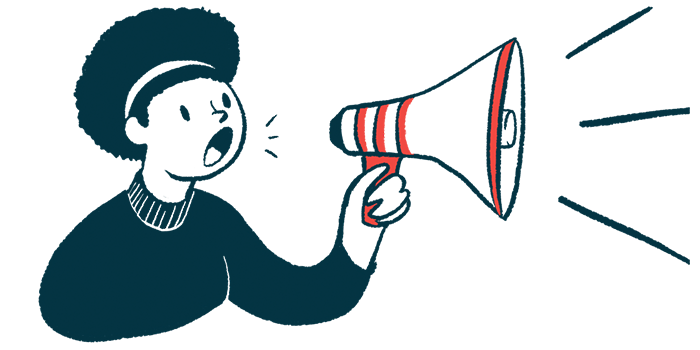
Analyzing patients’ voices using machine learning may help to identify Friedreich’s ataxia (FA) and differentiate it from other neurological disorders, a new study shows.
“The implications of this approach are substantial and provide new opportunities for healthcare, particularly for remote and rural areas where access to health providers might be limited,” researchers wrote.
The study, “Disease Delineation for Multiple Sclerosis, Friedreich Ataxia, and Healthy Controls Using Supervised Machine Learning on Speech Acoustics,” was published in IEEE Transactions on Neural Systems and Rehabilitation Engineering.
Voice changes are symptom of FA, other neurological disorders
Changes in voice are a characteristic symptom of FA. Many other neurological disorders, such as multiple sclerosis (MS) can also cause speech abnormalities as the nerves that normally control speech are damaged.
Theoretically, analyzing changes in patients’ voices could effectively identify and track the progression of neurological disorders like FA and MS, since vocal recordings can be collected painlessly and remotely using modern technology such as smartphones.
In recent years, many scientists have begun to explore whether machine learning could be used for vocal analysis in healthcare settings. Simply, machine learning involves feeding a bunch of data into a computer, alongside a set of mathematical algorithms that the computer uses to “learn” and identify patterns in the data.
Prior research has focused on differences in vocal recordings between specific patient populations, including FA and MS patients, and healthy people. In real-world practice, a computer would need to be able to distinguish not only between healthy people and people with a disease, but also between people with different diseases that share overlapping features.
In the new study, scientists expanded on previous research by creating a novel machine learning algorithm trained to distinguish between three groups: people with FA, people with MS, and healthy people.
“To the knowledge of the authors, this is the first paper to use machine learning to simultaneously differentiate three groups of disease classes … using speech data,” the researchers wrote.
These technologies promise to provide tools that can aid practitioners in reaching a diagnosis and relieve the physical and financial burden of patients.
1,000 recordings were included in study
The study included 73 people with FA, 112 with MS, and 229 without disease. For the study, participants conducted a vocal recording saying a set of defined syllables. Some individuals contributed multiple recordings so more than 1,000 total recordings were included.
Two-thirds of the recordings were used to train the computer learning algorithm, and then the remaining third was used to test the algorithm.
To test the algorithm’s accuracy, the researchers used a statistical measure called the area under the receiver operating characteristic curve, or AUC. This is a mathematical measure of how well a test can distinguish between two groups, such as people with or without FA. AUC values range from 0.5 to 1, with higher numbers reflecting better accuracy.
Results showed that the voice-based machine learning algorithm had a very high AUC of 0.98 for identifying FA. The AUC for identifying MS was 0.96, and for healthy controls, it was 0.97.
“These values indicate outstanding discrimination by the model,” the researchers wrote, adding the results “indicate that multiclass supervised machine learning has the potential to discriminate between diseases, a step beyond mere healthy-pathological dichotomies.”
In analyzing the model closer, the researchers found some distinguishing features the computer identified. For example, people with FA tended to have more variability in their voices and tended to take longer to speak each syllable.
Several features ‘strongly contributed to distinguishing between groups’
“We identified several acoustic features that strongly contributed to distinguishing between groups,” the scientists wrote.
Future studies might look into these features in more detail to determine exactly what parts of voice are most important to analyze when identifying diseases, the researchers added, noting that expanding the algorithms to include other data alongside vocal analyses might further improve the model’s accuracy. The model could be further expanded to include more disease states as more large datasets become available.
“Big data initiatives that bring together researchers and speech data from multiple laboratories are necessary to increase the scope of diseases that can be identified by acoustic clinical markers and machine learning. Moreover, a combination of remote testing tools for physical and cognitive assessment could be included in addition to speech to improve identification accuracy,” the scientists wrote.
“These technologies promise to provide tools that can aid practitioners in reaching a diagnosis and relieve the physical and financial burden of patients,” they concluded.