Specialized motion-capture suits may help predict FA progression
Suits could improve efficiency of clinical trials for slowly progressing diseases
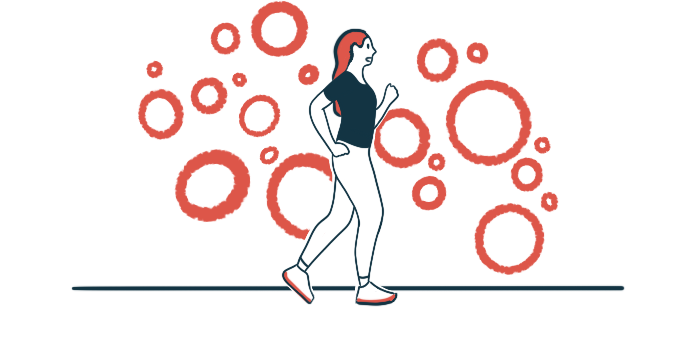
Motion-capture suits can be combined with machine learning to predict how Friedreich’s ataxia (FA) will progress, a new proof-of-concept study shows.
The technology may help to improve the efficiency of clinical trials in FA and other slowly progressing diseases, according to researchers.
The study, “A wearable motion capture suit and machine learning predict disease progression in Friedreich’s ataxia,” was published in the journal Nature Medicine.
FA trials have typically relied on clinician assessments
Clinical trials are the gold standard for testing whether or not potential treatments are effective. A key aspect of trial design is determining how exactly to measure efficacy.
FA trials have generally relied on standardized assessments of function like the Spinocerebellar Ataxia Functional Index (SCAFI) and Scale for the Assessment and Rating of Ataxia (SARA), which involve having a clinician assess a patient doing several set tasks.
Since they rely on clinician assessments, these scores are generally not very precise and can be subjective. This can pose a major challenge for trials: without a sensitive measure, it can be hard to identify changes, which can often take many months or years to be clinically measurable.
This means that trials usually have to go on for years to have any chance of detecting an effect. The lack of sensitive measures also means that trials have to include many participants for the results to be statistically meaningful — a major challenge for rare diseases like FA.
In this study, a team of scientists in the U.K. investigated whether using full-body motion-capture suits could be used to improve the precision of FA tracking.
The study included nine people with FA and an equal number of controls without any known disease. Participants underwent assessments including the SCAFI and SARA at an initial visit (baseline), then again after three weeks, three months, and nine months.
Our suit features contained sufficiently rich information not only to score the disease state of the patient in the present but also to predict how the disease would evolve.
During these assessments, they wore the motion-capture suits, which collected detailed data about their movements. The researchers specifically focused on data collected from two parts of the SCAFI, the eight-meter walk test (which measures walking ability) and the nine-hole peg test (which measures arm and hand dexterity).
Of note, one of the FA patients included in the study was not able to do the walking test, but was still able to be included for analysis of arm dexterity scores.
“This establishes that our methodology can be used to predict the clinical scales for nonambulatory patients too,” the researchers wrote, noting that trials often exclude nonambulatory patients because it can be hard to measure disease progression in these individuals using standard measures.
Using the collected data, the researchers defined several kinematic features — that is, defined measures of body movement during the tasks.
“Our features quantify the complex and subtle movement patterns that may escape even very experienced clinicians,” the researchers wrote, adding “the high accuracy of the collected data can also be used to better understand the subtle changes in behavior secondary to the disease or ameliorated by therapeutic intervention.”
The team used machine learning to assess whether analysis of the features at baseline could be used to predict SCAFI and SARA scores nine months later.
Results showed that, generally, the kinematic features showed strong predictive associations with future disease scores. The coefficient of determination (R2) — a measure of variation that can range from 0 to 1, with higher scores reflecting better outcome prediction — was generally around 0.8, indicating little variation between predicted scores and the actual scores.
“This implies that our suit features contained sufficiently rich information not only to score the disease state of the patient in the present but also to predict how the disease would evolve,” the researchers concluded.
Technology could shorten clinical trials, reduce number of patients required
FA is caused by a mutation in the FXN gene, leading to less production of FXN messenger RNA (mRNA) and, ultimately, reduced levels of the frataxin protein. Further analyses showed that the suit-derived kinematic features showed significant associations with the level of FXN mRNA in patients’ cells. Meanwhile, scores on the SCAFI and SARA did not show strong associations with FXN mRNA levels.
This suggests that the kinematic features “carry crucial information about the disease and can directly link behavior with FXN gene expression,” the researchers wrote.
While the scientists stressed that more research is needed to expand on this approach and validate the findings of this small study, they said that using this type of technology to measure disease progression “promises to shorten clinical trials that would otherwise be prohibitively long or reduce the number of patients required,” which “is especially important in rare neurodegenerative diseases.”
The researchers also noted that the kinematic features aren’t reliant on any particular type of sensor — instead, they are based on analyses of the body’s position in space. Theoretically, this data could be acquired from video, though current technology cannot do it very accurately.
“We believe that as technology progresses for the accurate skeletal reconstruction of human movement from video, remote clinical evaluation of patientsʼ motor performance, even in a home setting, will become possible,” the researchers wrote.