Wearable sensors may help monitor FA disease severity in daily life
Researchers evaluated use of sensors for monitoring activity in 39 patients
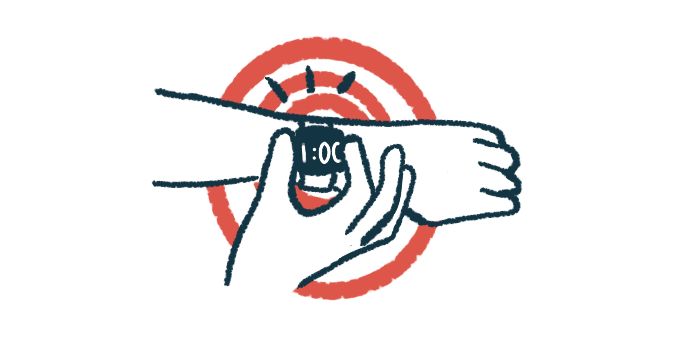
Data from wearable sensors that are designed to collect real-world information about a person’s ability to function in daily life correlated well with standard clinical assessments of disease severity among people with Friedreich’s ataxia (FA), a study finds.
These types of remote monitoring tools could help to better track a patient’s functional status in his everyday environment and offer a more detailed picture of disease severity that can support clinical trials and routine patient care, the researchers said.
“Our results establish the initial clinical validity of using wearable sensors in assessing disease severity and monitoring motor dysfunction in [FA],” they wrote. The study, “At-home wearable-based monitoring predicts clinical measures and biological biomarkers of disease severity in Friedreich’s Ataxia,” was published in Communications Medicine.
In FA, worsening muscle control and coordination, called progressive ataxia, result from a loss of the frataxin protein due to mutations in the FXN gene. Over time, patients experience gait impairments, loss of upper limb coordination, and speech difficulties, among other symptoms.
When new treatments are tested in clinical trials, they’re often assessed on their ability to slow disease progression, as evaluated by clinical tests such as the modified Friedreich’s Ataxia Rating Scale (mFARS). But this assessment has limited sensitivity to detect changes in patients’ clinical status. Moreover, because the data is collected on a set schedule at the clinic, it doesn’t adequately capture how patients function in real-world situations and how symptoms fluctuate day to day.
Remote technologies that are capable of monitoring patients at home are gaining traction as a way of collecting more routine data from people with chronic diseases in their daily lives. These include wearable sensors that can continuously collect information about how a person is moving around in their home environment.
Monitoring daily activity remotely
Here, researchers examined the utility of wearable sensors for monitoring disease severity in 39 FA patients recruited as part of a larger FA natural history study (NCT06016946).
All the participants were monitored over a week, with the analysis only considering the days the sensors — a PAMSys pendant worn on a lanyard around the neck to gather information on walking and moving around, and a PAMSys ULM wrist sensor for assessing upper limb movements — were worn at least 18 hours.
Each patient also underwent a standard in-clinic assessment, where a neurologist evaluated them using the mFARS and other functional tests.
Most participants (31 of 39) wore the neck sensor exactly as they were asked to, while eight had a compliance of about 95%. Similarly, all but two people wore the wrist sensor with 100% compliance, while two people wore it with 90% compliance.
“This signifies that [FA] patients are consistently and effectively using these devices and at-home monitoring of their physical activity and movement patterns using wearable sensors is feasible in [FA],” the researchers wrote.
Physical activity metrics collected from the sensors significantly correlated with clinical (e.g. mFARS) and biological measures (e.g. frataxin levels, GAA mutation), results showed. For example, locomotion metrics such as total walking time and step frequency had significant negative correlations with scores on mFARS Section E, which evaluates upright stability. Such findings indicate greater walking ability, as captured by the sensors, is associated with less impairment in clinical assessments.
Other correlations reflected a similar pattern. In particular, features of goal-directed movements such as those related to velocity and acceleration also significantly correlated with clinical scores and frataxin levels.
With the information collected from the sensors, the scientists developed artificial intelligence-powered models for predicting FA disease severity.
A model that took into account multiple types of sensor data along with other demographic information, disease duration, and FXN mutation data had the strongest ability to predict clinical aspects of disease severity.
“These findings highlight the potential advantages of a multimodal approach to disease assessment and its role in enhancing the prediction of disease severity,” wrote the researchers, who noted that a lack of long-term data from the participants limits the ability to look at how disease progression changes over time. “Future research initiatives should focus on validating our technology and methodology through a longitudinal study involving a broader and more diverse participant cohort.”